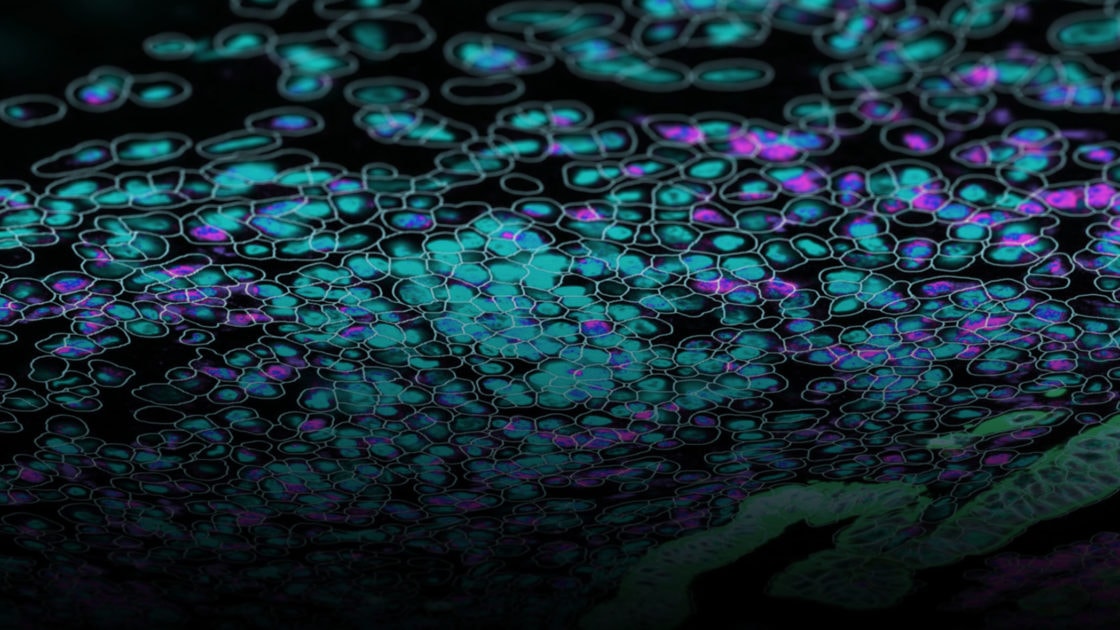
Situating Single Cell RNA-seq in the Context of Multiomics
Cells, the building blocks of all life, are built from biological molecules such as proteins and RNA. Biological molecules perform all the functions of living cells, from DNA replication to intercellular communication. Understanding cells thus requires understanding biological molecules and their functions, particularly at the individual cell level.
Many methods are available for assessing each type of molecule in cells – DNA, RNA, protein, sugars, lipids. Until recently, analyses of biological molecules required thousands, if not billions, of cells from a tissue to obtain the absolute quantity needed to detect the molecule of interest. Newer technology is now capable of detecting the very small amounts of a given molecule in a single living cell.1Haque A, Engel J, Teichmann SA, Lönnberg T. A practical guide to single-cell RNA-sequencing for biomedical research and clinical applications. Genome Med. 2017 Aug 18;9(1):75. doi: 10.1186/s13073-017-0467-4. PMID: 28821273; PMCID: PMC5561556.
The techniques used to detect biological molecules from a single cell depend on the type of molecule being detected.
Changes in cellular programming typically involve modification of gene expression, for which the initial step is RNA production. Detection of RNA is therefore one of the most common procedures needed for studying cellular biology. Although detecting RNA (and DNA) from tissues usually involves hybridization of probes to target sequences, detecting RNA at the single cell level has traditionally used nucleic acid sequencing techniques, typically in combination with some form of PCR to amplify targets for enhanced detection.
Quantitative Real Time Polymerase Chain Reaction (qRT-PCR or qPCR) and RNA-sequencing (RNA-seq) have both been adapted for use on transcripts from single cells. Single cell RNA-seq (scRNA-seq) has been particularly useful for analyzing transcriptomes of single cells.
This article answers questions about scRNA-seq and discusses newer techniques that accelerate understanding of cell biology by adding spatial context to a cell’s transcriptomic profile.
What is quantitative polymerase chain reaction?
A key concept underlying scRNA-seq is qPCR, which provides quantitative information about gene expression in cells of interest. After isolating RNAs and converting them into a cDNA library using reverse transcriptase, primers for genes of interest are used to amplify specific genes (known as rt-PCR). Fluorescently labeled nucleotides incorporated during each round of PCR are read and quantified by the qPCR machine. In this way, qPCR quickly obtains gene expression information for quick, easy analysis that does not require sophisticated bioinformatic techniques, unlike RNA-seq (discussed below).
As a result, it is a commonly used technique for gaining insight into gene expression changes during processes such as cell signaling cascades after agonist treatment in knock-out versus wild type cell lines. However, qPCR use is limited to known genes, and is not useful for discovering novel genes or characterizing different RNA species.
What is RNA-sequencing?
RNA-seq uses next-generation sequencing (NGS) to understand global transcriptional dynamics in a cell, and therefore does not require knowledge of gene targets prior to an experiment. In contrast to qPCR, which can only quantify transcripts of known genes, RNA-seq can be used to identify novel transcripts and quantify transcripts present in a tissue. RNA-seq can examine only coding RNAs, only non-coding RNAs, or both, depending on how the cDNA library is constructed. For example, coding RNA species are screened using a cDNA library constructed with a primer that recognizes mRNA poly-A tails prior to a reverse transcriptase (rt) step. cDNAs in the library are then indexed with a barcoding system for tracking NGS results. Library construction also allows researchers to study splice variants and other post-transcriptional modifications, transcriptional dynamics before and after drug treatments, and gene expression analysis in different cell types, tissue context, or healthy versus diseased tissues.
The range of applications for RNA-seq spans every area of biology where gene expression analysis can provide insights, but it lacks information about the identity of the cells expressing any given gene.
The range of applications for RNA-seq spans every area of biology where gene expression analysis can provide insights.2Han, Y., Gao, S., Muegge, K., Zhang, W., & Zhou, B. (2015). Advanced applications of RNA-sequencing and challenges. Bioinformatics and biology insights, 9, BBI-S28991. However, since the source material is whole tissue, RNA-sequencing lacks information about the identity of the cells expressing any given gene. Techniques for sequencing RNA in single cells is therefore required for elucidating cell type-specific expression. scRNA-seq (see below), a subtype of RNA-sequencing, gives transcriptomic data from single cells that builds on the information provided by RNA-seq. Gene expression profiles involved in processes ranging from alternative splicing to effects of single nucleotide polymorphisms and disease states can be examined with scRNA-seq and used to guide research forward.
What is single-cell sequencing?
As the name implies, single-cell sequencing is a method of obtaining DNA or RNA-sequence information from single cells. Single cells are isolated after tissue dissociation, and either DNA or RNA is purified for library construction. Library sequence information is then analyzed, providing insight into gene expression profiles and cellular functions for specific cell types. Single-cell DNA sequencing is useful for identifying mutations in individual tumor cells,3Li Y, Jiang X, Zhong M, Yu B, Yuan H. Whole Genome Sequencing of Single-Circulating Tumor Cell Ameliorates Unraveling Breast Cancer Heterogeneity. Breast Cancer (Dove Med Press). 2022 Dec 28;14:505-513. doi: 10.2147/BCTT.S388653. PMID: 36597488; PMCID: PMC9805725. for example, while scRNA-seq uses are mentioned below.
Single-cell sequencing of DNA and RNA expands understanding of cell functions, even though any three-dimensional spatial context is disrupted by tissue dissociation.
What is single cell RNA-seq?
A combination of RNA-seq and single-cell sequencing, the main difference between scRNA-seq and RNA-seq is the source of RNA used. Both follow similar technical methods (i.e., RNA isolation, library construction, etc), but scRNA-seq is performed on transcripts from a single cell or a distinct population of cells, while conventional RNA-seq uses transcripts aggregated from multiple cell types (i.e., whole tissue). The information derived from each is therefore qualitatively different. Batch sequencing performed by RNA-seq provides an overview of transcriptional dynamics in a tissue, while scRNA-seq is performed on isolated cells sorted into biologically interesting populations. Otherwise, library preparation and sequencing methods are essentially the same between the two techniques.
Using scRNA-seq, investigators can assess biological differences between distinct cell types.
Using scRNA-seq, investigators can assess biological differences between distinct cell types, thus gaining information about specific cells or subpopulations of cells lost in batch sequencing. scRNA-seq is particularly valuable for understanding biologically important but uncommon cells such as stem cells or circulating tumor cells,4Baccelli, I., Schneeweiss, A., Riethdorf, S., Stenzinger, A., Schillert, A., Vogel, V., … & Trumpp, A. (2013). Identification of a population of blood circulating tumor cells from breast cancer patients that initiates metastasis in a xenograft assay. Nature biotechnology, 31(6), 539-544. and can also assess changes in these cells over time during a process such as differentiation. For example, characterizing the transcriptional shifts that occur during differentiation can lead to identification of lineage-specific stem cell markers.5Trapnell, C., Cacchiarelli, D., Grimsby, J., Pokharel, P., Li, S., Morse, M., … & Rinn, J. L. (2014). The dynamics and regulators of cell fate decisions are revealed by pseudotemporal ordering of single cells. Nature biotechnology, 32(4), 381-386. scRNA-seq information such as this may lead to a better understanding of how to stimulate tissue regeneration after an injury, or, in the context of diseases, may help researchers understand why some cancers return after a period of remission. The ability of scRNA-seq to capture and sequence rare cells will provide insights into a variety of disease processes, leading to improved treatments.6Saliba, A. E., Westermann, A. J., Gorski, S. A., & Vogel, J. (2014). Single-cell RNA-seq: advances and future challenges. Nucleic acids research, 42(14), 8845-8860.
New technologies enhance the information derived from scRNA-seq
Recent technologies improve on information gained from scRNA-seq to provide a robust analysis of gene expression at the single-cell level. Known as spatial transcriptomics, these techniques, unlike scRNA-seq, give cell type-specific gene expression information within a cell’s native tissue context.
With the capability to provide protein and RNA information in a cell type, spatial transcriptomics preserve the spatial information to provide a fuller understanding of the cell’s biological function. Performed on either formalin-fixed paraffin embedded (FFPE) or fresh frozen tissue samples mounted on glass slides, spatial transcriptomics use fluorescently labeled in situ hybridization probes or antibodies, depending on the molecule of interest. Up to 1000 RNA transcrips and over 100 protein targets can be analyzed in a single experiment. Simultaneous visualization of proteins and RNA allows for distinguishing cell boundaries and identifying cell types.
Requiring fewer steps than scRNA-seq techniques, spatial transcriptomics is more robust with higher sensitivity for detecting low-expressing RNA or protein.
The in situ hybridization techniques used in spatial transcriptomics do not require cDNA library preparation, but instead detect target molecules with barcoded probes that can be analyzed by bioinformatic techniques. Requiring fewer steps than scRNA-seq techniques, even those with spatial context capabilities, spatial transcriptomics is more robust with higher sensitivity for detecting low expressing RNA or protein.
Spatial transcriptomics has many applications, including generating cell type expression atlases and identifying pertinent biomarkers. Once established, atlases and biomarkers can serve as a baseline for examining healthy versus diseased tissues, or tissue response to various stimuli. For example, biomarker analysis could ascertain changes in a tumor in response to chemotherapy treatment. The combination of gene expression and spatial data derived from spatial transcriptomics provides exponentially more biological information than scRNA-seq alone.
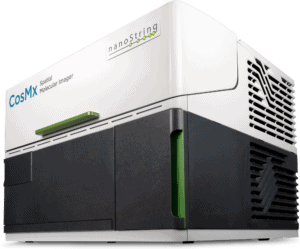
Since cellular processes are responsible for maintaining tissue structure and function, spatial biology analysis can characterize the ways cells interact and communicate during those processes. Cell signaling, such as ligand-receptor interactions, is a key component of cell-cell interactions. A ligand binding to its receptor triggers a cascade of biological processes in a cell, often culminating in changes in gene expression. Spatial transcriptomics can reveal what happens in these cascades before, during, and after ligand binding.
NanoString has developed a spatial transcriptomics platform with single cell resolution. The CosMx™ Spatial Molecular Imager (SMI) visualizes and quantifies RNA and protein at the single cell and subcellular levels. The associated bioinformatics platform, AtoMx™ Spatial Informatics Platform (SIP), analyzes the results from CosMx SMI.
The CosMx™ SMI and decoder probes are not offered and/or delivered to the Federal Republic of Germany for use in the Federal Republic of Germany for the detection of cellular RNA, messenger RNA, microRNA, ribosomal RNA and any combinations thereof in a method used in fluorescence in situ hybridization for detecting a plurality of analytes in a sample without the consent of the President and Fellows of Harvard College (Harvard Corporation) as owner of the German part of EP 2 794 928 B1. The use for the detection of cellular RNA, messenger RNA, microRNA, ribosomal RNA and any combinations thereof is prohibited without the consent of the President and Fellows of Harvard College (Harvard Corporation).
How is spatial transcriptomics better than scRNA-seq?
scRNA-seq improves on RNA-seq by performing more refined gene expression analysis. Unlike qPCR or RNA-seq, scRNA-seq generates RNA-sequence data from specific cells of interest instead of batches of collected cells. In addition, scRNA-seq and RNA-seq can be used to identify and explore previously unknown genes or RNA species since they do not require gene specific primers. However, dissociation of tissues into individual cells for analysis destroys spatial context information pertinent to understanding cellular function.
Spatial transcriptomic techniques, such as CosMx SMI, add to the sequencing information of scRNA-seq by including the spatial context of the cells of interest.
Spatial transcriptomic techniques, such as those used by CosMx SMI, add to the sequencing information of scRNA-seq by including the spatial context of the cells of interest. Furthermore, spatial transcriptomics can also provide proteomic information, making it a true spatial multiomic platform. Visualization and quantitation of both RNA and protein on the same tissue samples, in a spatial context, provides significant information regarding the interactions and functions of cell types in a tissue. This is important for identifying differences under certain conditions such as drug treatment or disease state. In this way, spatial transcriptomics surpasses scRNA-seq.
Connecting scRNA-seq with spatial transcriptomics
While every technology has its own benefits and challenges, scRNA-seq and spatial transcriptomics can be used in conjunction with one another. Insights about cell-type specific gene expression gained from scRNA-seq can be used to inform experiments using CosMx SMI.
For example, a 1000 plex gene expression panel developed at NanoString for CosMx SMI facilitates the mapping and profiling of individual cells, as well as examining cell-cell interactions. Using a combination of publicly available scRNA-seq data and CosMx SMI data, researchers were able to map cell types across tissues. This ability to connect gene expression with spatial location in a tissue has implications regarding specific cellular functions that may prove critical for deciphering specialized microdomains within cells, and thus directing related research into basic biology and disease-related research.
References
- 1Haque A, Engel J, Teichmann SA, Lönnberg T. A practical guide to single-cell RNA-sequencing for biomedical research and clinical applications. Genome Med. 2017 Aug 18;9(1):75. doi: 10.1186/s13073-017-0467-4. PMID: 28821273; PMCID: PMC5561556.
- 2Han, Y., Gao, S., Muegge, K., Zhang, W., & Zhou, B. (2015). Advanced applications of RNA-sequencing and challenges. Bioinformatics and biology insights, 9, BBI-S28991.
- 3Li Y, Jiang X, Zhong M, Yu B, Yuan H. Whole Genome Sequencing of Single-Circulating Tumor Cell Ameliorates Unraveling Breast Cancer Heterogeneity. Breast Cancer (Dove Med Press). 2022 Dec 28;14:505-513. doi: 10.2147/BCTT.S388653. PMID: 36597488; PMCID: PMC9805725.
- 4Baccelli, I., Schneeweiss, A., Riethdorf, S., Stenzinger, A., Schillert, A., Vogel, V., … & Trumpp, A. (2013). Identification of a population of blood circulating tumor cells from breast cancer patients that initiates metastasis in a xenograft assay. Nature biotechnology, 31(6), 539-544.
- 5Trapnell, C., Cacchiarelli, D., Grimsby, J., Pokharel, P., Li, S., Morse, M., … & Rinn, J. L. (2014). The dynamics and regulators of cell fate decisions are revealed by pseudotemporal ordering of single cells. Nature biotechnology, 32(4), 381-386.
- 6Saliba, A. E., Westermann, A. J., Gorski, S. A., & Vogel, J. (2014). Single-cell RNA-seq: advances and future challenges. Nucleic acids research, 42(14), 8845-8860.