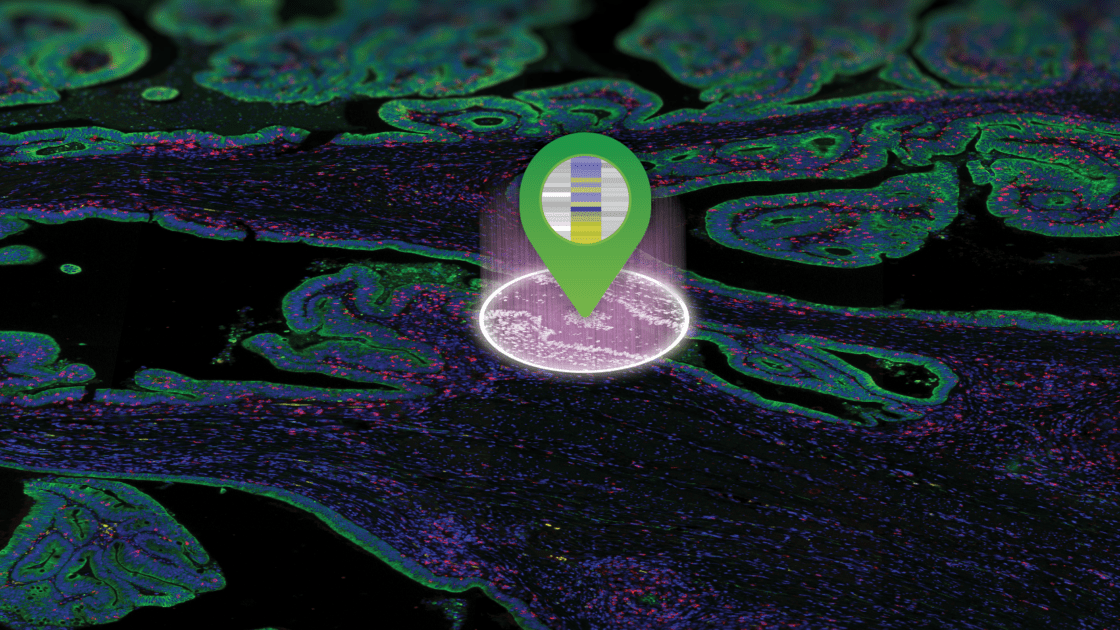
The Birth of Spatial Genomics
NanoString catalyzed the spatial biology revolution in 2019 with the launch of the GeoMx® Digital Spatial Profiler (DSP), but did you know that the beginnings of this nascent field go all the back to the early 1990s with the idea to spatially map tissue structure and function in embryonic development? In fact, the first patent for spatial biology that coined the term Spatial Genomics was filed in the year 2000 and was finally issued in 2009. We spoke to Mike Doyle, Ph.D., Vice President of Research at the New Mexico Institute of Mining and Technology who is one of the co-inventors of spatial genomics about those heady early days and what led him to start thinking about spatial biology.
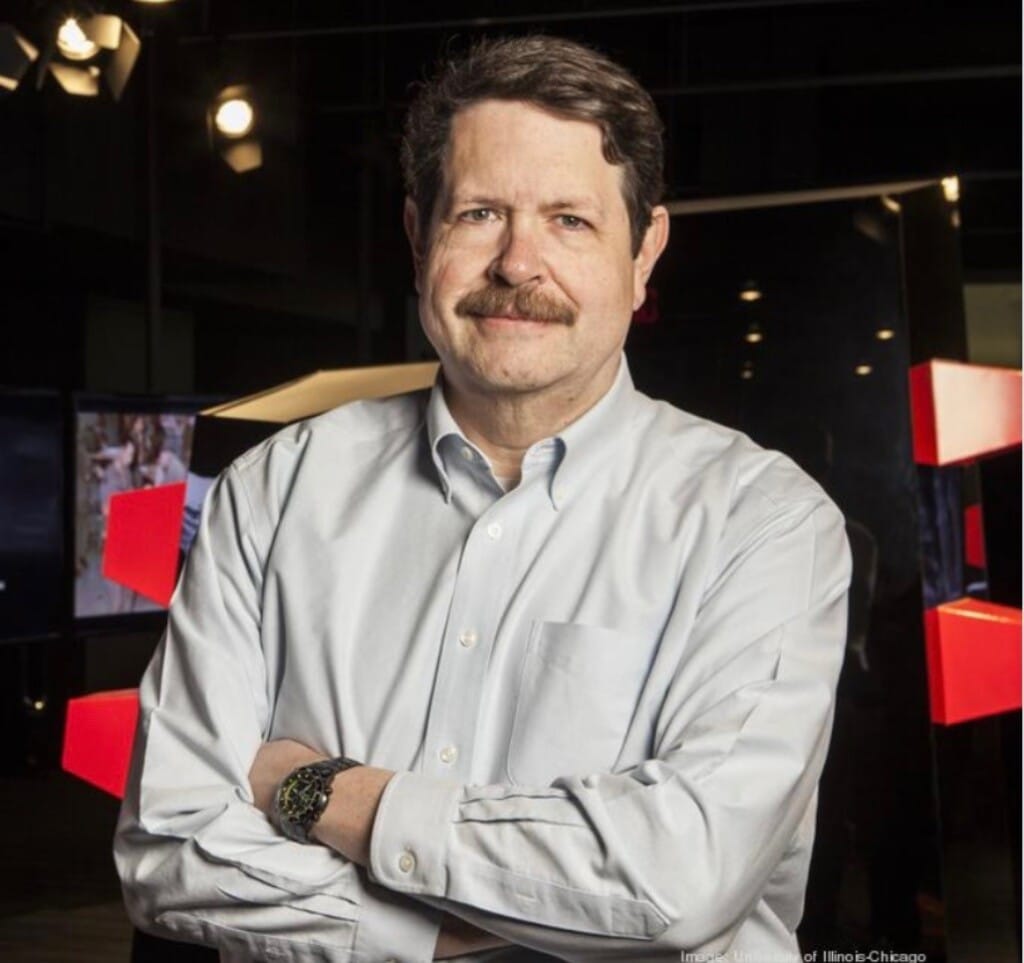
The Early Days Before Spatial Genomics
NSTG: Hi Mike! Thanks so much for joining us for a Q&A interview on the NanoString Blog! Can you tell us a little bit more about your background for our readers?
Mike Doyle: In the mid-1980s, I was working as a medical illustrator for the Pritzker School of Medicine at the University of Chicago when I decided to go back to school to get a Ph.D. in anatomy and cell biology.
In grad school, the lab I was working in had a sophisticated (for the times) image analysis system, and I decided to see if I could use it in some way to help in the histology class I was teaching. I thought that if I could find a way to make the microscopy images interactive, I could use it as the basis for building an automated histology teaching assistant for medical students.
I came up with a way to encode semantic object identity into the internal representations of image pixel data, essentially turning every image pixel into an independently addressable hotspot. This allowed me to create an early distributed hypermedia system, where a small browser app could traverse links in images to related textual, image, programmatic, etc. media, allowing the user to browse a knowledge web of virtually unlimited size.
This early success got me hooked on trying to find ways to use computer imaging technology to help in the study of biomedical subjects. Another class I was involved in at the time was vertebrate embryology. A typical lab activity in the class was for the students to look at a series of sequential serial sections of frog, chick and pig embryos through a dissecting microscope and try to reconstruct and visualize in their minds the three-dimensional structure of the embryo to gain an understanding of the developing shape of the growing embryonic structures. Some students could do this easily, maybe the ones who went on to become great surgeons, but most found it a difficult task.
It occurred to me that, if I could write a computer program to stack those cross sections into a 3-dimensional structure we would have a valuable tool to enable all the embryology students to be able to see how the embryonic structures emerged and related to each other, and study those shapes from a variety of vantage points. I started a project, collaborating with the course director, Maury Pescitelli, to begin developing a computer-aided embryology visualization system.
Our lab had just purchased IBM’s ‘powerful’ new AT personal computer, which we thought would be more than powerful enough for the job I had in mind. After many months of discovering how complex the problem we had undertaken actually was, and how underpowered that machine was for the task at hand, I was saved by the bell, or in this case, by being recruited to join the faculty of the newly redesigned Department of Biomedical Visualization at the University of Illinois at Chicago.
NSTG: How did you get involved in the Biomedical Visualization Laboratory at the University of Illinois, Chicago (UIC)?
Mike Doyle: This newly remade department at UIC was headed by Lew Sadler, an extremely innovative medical illustrator who had become deeply involved in computer imaging and visualization when he co-created the first system for age-progression of photographs of missing children, for the National Center for Missing and Exploited Children. Lew visited me in Urbana, to recruit me to join the department, and did a great job of selling the vision of a new department devoted to imaging science and visualization of biological phenomena.
After moving to the Chicago campus, I became the Director of the UIC Biomedical Visualization Laboratory, a campus resource for technology development and tech transfer relating to biomedical imaging and applications. One of the goals of the lab was to create a set of canonical models of anatomical structure, with the aim of using those models as the basis to build knowledge bases that could integrate both functional and structural information about organisms.
At around the same time, I was appointed to the oversight committee for a new project from the National Library of Medicine, called the Visible Human Project. The goal of the project was to create a national resource for ultra-high-resolution 3D image data on adult human anatomy. I was extremely interested in working with this kind of information, but it would be several years before any of the Visible Human data was going to become available.
NSTG: What was the Visible Embryo Project (VEP)? How did you get involved?
Mike Doyle: I didn’t want to wait for the Visible Human Project data to become available, so I began looking for other sources for high resolution 3D images of human anatomy.
I discovered a collection at the National Museum of Health and Medicine called the Carnegie Collection of Human Embryology. This collection is a set of about 650 human embryos, serially sectioned and mounted on slides that were stored at the museum in DC. It reminded me very much of the embryo stacking project I had attempted while in grad school.
I realized that, if I could come up with a way to digitize and stack those sections, I’d essentially have a set of image data comparable to 650 Visible Human Projects. I connected with Adrianne Noe, the Director of the Human Developmental Anatomy Center at the museum, which had just taken possession of the collection, and she was enthusiastic about the potential of such an effort. So, I started working on building the tools necessary for making it possible.
During most of 1992, my group, including an extremely talented graduate student named Cheong Ang, worked on solving various problems involved with digitizing and aligning the Carnegie embryo data, creating enormous datasets of multidimensional image information. One of the problems we encountered was finding powerful enough computational resources to allow the 3D visualization of such large volumes. Luckily, I had begun collaborating with Ingrid Carlbom and Demetri Terzopoulos at the Digital Equipment Corporation’s Cambridge Research Lab on using high-performance computing to reconstruct and visualize these types of data, and they provided access to some of their high-power computing resources, as well as valuable tools for aligning the image data.
By the summer of 1992, we were able to do a demonstration at SIGGRAPH, in Chicago, of an embryo volume reconstruction rendered in real time on a 64 thousand processor DEC Maspar supercomputer and viewed on a Silicon Graphic Iris workstation. Quite a step up from the IBM AT I had begun my journey with several years earlier.
One of the major problems we encountered with 3D reconstructions of microtome-generated serial sections through biological tissue was the issue of dimensional stability. While the sections in the Carnegie Collection were expertly created, it was impossible for the original embryologists who had made the sections to prevent spatial artifacts from being introduced by the process. Each section was like a little rubber sheet that would be compressed or stretched in one or more dimensions by the forces involved in creating the slices and mounting and staining the tissue. A lot of our image processing work became involved with using various computational strategies to correct for these spatial artifacts.
Because of this, I started to become interested in other ways to image that kind of data. In addition to the serial-sectioned specimens, the collection also contained several intact embryos. I wondered if there might be a way to capture high-resolution 3D volume data of the specimen morphology from the intact embryo specimens using noninvasive technologies, such as tomographic imaging of one kind or another.
I reached out to a friend I had met while I was a graduate student in Urbana, Paul Lauterbur, who had created the field of magnetic resonance imaging years before in his lab at Stony Brook University in New York. Of course, he would later go on to receive the 2003 Nobel Prize for his invention of MRI. But back in the ‘80s, Paul had been recruited by UIUC and had moved to Urbana at about the same time I had begun my graduate school career. Word had gotten out around campus that I had become skilled in microscopic image processing, and Paul needed some help with image processing for some magnetic dipole experiments he was conducting.
I became friends with Paul’s graduate student, and we worked together for a while around 1987 on a side-project idea I had for reconstructing the 3D thickness of transmission electron microscopy sections by tilting the TEM section stage and capturing a series of ultrastructure images at varying angles, with the aim of digitizing those images and using back-projection tomography to reconstruct the 3D volumes of the TEM tissue sections. I can’t remember why, but after I created and gave him several sets of tilt-images, we never completed that side-project. I think our advisors must have suggested our time would be better spent working on our dissertation projects, though time has proven that unfortunate, since other researchers used the same approach many years later to develop what’s known today as Electron Tomography.
Returning to 1992, I reached out to Paul to ask if he had any interest in microscopic MRI imaging of embryo specimens, and he enthusiastically said yes. He had been doing a lot of microscopic MRI experimentation, and thought trying it on the historic embryo specimens would be an interesting challenge. So, in the fall of 1992, I did a sabbatical in Urbana, to work in Paul’s lab on novel magnetic coil designs for acquiring high-resolution embryo MRI datasets.
The US government had recently initiated what was called the High-Performance Computing and Communications (HPCC) Initiative, which was headed by Donald Lindberg, then the Director of the National Library of Medicine. I became excited about the possibility of putting together an HPCC project based on the Carnegie Collection data, and while I was working in Paul’s lab, I began discussing possibilities with Adrianne Noe on the phone, and spent the evenings at the Espresso Royale coffee shop in Urbana, writing the plan for a large multi-institutional project I named the Visible Embryo Project.
The main objective for the project, as I described in the original plan, was “to develop software strategies for the development of distributed biostructural databases using cutting-edge technologies for high-performance computing and communications (HPCC) and to implement these tools in the creation of a large-scale digital archive of multidimensional data on normal and abnormal human development.”
An interesting bit of trivia is that I found out years later that I had been working those nights in that coffee shop only a table or two away from Mark Andreessen and Eric Bina who were working on the first version of the NCSA Mosaic Web browser in the same coffee shop at the very same time.
The First Talks Around Spatial Genomics
NSTG: How did you and your colleagues come up with the idea of spatial genomics?
Mike Doyle: An ongoing theme of my lab at UIC was to build biostructural knowledge bases that could integrate both functional and structural information about various organisms, so one of the major goals of the Visible Embryo Project from the very beginning was to come up with ways to map biological function data onto multidimensional images of embryo specimens, to essentially create maps of genomic activity within tissue morphological context.
In fact, a video clip survives from a presentation I gave in January of 1994 in the corporate briefing center at Silicon Graphics Corporation where I describe this objective, describing to the audience: “We’re also looking at using these models as a basis for creating three-dimensional maps of gene expression, which is a way to correlate the findings of the Human Genome Project within a context that everyone uses. It sort of sets up a standard space within which everyone can report their findings, so that you can finally have some way of comparing studies that happen in different laboratories. If you’re studying the three-dimensional distribution of a gene relating to heart development, what you do now is you have a little fluorescent marker that glows under an ultraviolet light, and you use confocal microscopy to develop a three-dimensional model of it, and then you say, well it’s on here and it’s on there and you try to describe it in anatomical terms but it is a qualitative description, right? But here there would be a standard anatomy space that people could use to describe their findings so that they could, rather than say, yeah we saw five different studies that said this was expressed at the bifurcation of the Aorta with the Common Carotid artery — you don’t have to do it in terms of verbal descriptions, you can do it in terms of a true, measurable Cartesian coordinate system.”
In the late 1990s, I worked with three VEP collaborators, Maury Pescitelli, of UIC, Betsey Williams, of Harvard, and George Michaels, of George Mason, to design a way to achieve that goal, creating a system to enable the multidimensional mapping of gene expression within tissue morphological context, something we called “Spatial Genomics.” We filed the first provisional U.S. Patent application for that system on July 28, 2000. The related patent eventually issued on November 3, 2009 (7,613,571).
The Technologies That Started It All
NSTG: Can you tell us a little bit about the first technology that was developed to do spatial genomics? How did it work?
Mike Doyle: Our system, named SAGA, for Spatial Analysis of Genomic Activity, involved the basic steps of generating a 3D morphological rendering of a tissue sample, subsampling the tissue along a regular grid pattern, bar-coding each subsection sample, analyzing each sample for gene expression, and then mapping the gene expression data back onto the morphological rendering of the tissue.
Various methods could be used to accomplish the tissue subsampling. One example we showed involved using sets of alternating serial sections, staining one set for microscopic imaging, and using the alternating set for what we called “tissue rasterization,” which involved incising a regular grid pattern across each tissue section using a laser, and then robotically isolating each square of the grid, each tissue subsample, to a uniquely coded isolation tube for lysis and further processing. Each tube would be bar coded to indicate the x,y,z tissue space coordinate of the original morphological matrix location of the sample.
Each subsample would then go through RNA amplification using reverse transcription, PCR, and cDNA microarray analysis. Computer-based image acquisition, processing, and analysis would then be used to quantify the strength of fluorescent signals from the microarrays and the resultant gene expression data would be mapped onto the original morphological matrices of image data. The resultant expression-annotated spatial reconstructions could then be used to elucidate biological function, multiplexing the visualization of the expression activity of an enormous number of genes within individual morphological reconstructions.
That initial patent specification described the foundation for what later became known as spatial transcriptomics and laid out a roadmap for others to follow in the future.
Over fifteen years passed, however, before Patrik Stahl and his collaborators in Stockholm, Sweden, picked up the technique and modified the tissue subsampling, coding and analysis steps, publishing a landmark 2016 paper in Science to widespread acclaim. Since then, spatial genomics has exploded in popularity and use throughout the bioscience community.
NSTG: Why is spatial genomics important for the study of biology?
Mike Doyle: Before the advent of spatial biology, investigators could study various aspects of the biological function of specimens, and they could separately study the precise morphological structures of those specimens, but those two activities were mutually exclusive of each other. In something akin to a biology analog of the Heisenberg Uncertainty Principle, the act of observing the functional aspects of a sample destroyed the ability to observe the fine structural detail. You could have one or the other, but never both at the same time. Spatial biology changed all that. As the old maxim states, “Context is King”, only by being able to visualize the precise spatial distribution of function-correlated signals, within the spatial morphological context of the tissue within which the functions occur, can investigators begin to unravel the detailed spatial interrelationships involved in both normal and abnormal biological functions. That’s now possible with spatial genomics.
NSTG: What excites you the most about the field of spatial genomics?
Mike Doyle: I’m extremely excited by the rapidity of the adoption and expansion of the paradigm across a wide range of biological application areas. It was immensely gratifying to see the method we created in the late ‘90s be named “Method of the Year” by Nature in January of 2021, over 20 years after our work. It’s so exciting to see almost daily reports of new discoveries that are being made using spatial transcriptomic approaches, and the amazing new tools being created by NanoString and others that are pushing the boundaries of spatial resolution, dimensionality, and richness of data to new extremes.
NSTG: You were also involved in the first use of the ‘cloud’ for analysis of data from the VEP. How important is data analysis in the cloud when it comes to spatial genomics?
Mike Doyle: The Visible Embryo Project started with an audacious goal: to make real-time interactive 3D volume visualizations of enormous high-resolution embryo datasets available to end-users over the commodity Internet. To put this into context it’s important to remember that, in 1992, the fastest Internet connections available to typical end-users was via dial-up modems, and the most powerful personal computers were several orders of magnitude less powerful than the least powerful computers available today. What we were attempting to do simply wasn’t possible with the technology available at the time. So, we had to invent entirely new technologies each step of the way.
An early problem was how to make the system easy to use by scientists from wherever they were. The recently launched NCSA Mosaic web browser presented an interesting possibility for a solution, but it was extremely limited. For people today used to using their browsers to easily access things like streaming video, online gaming, social networks, and online shopping, it may be hard to remember that, back in the early 1990s, the Web was extremely primitive. Even Microsoft’s 1994 home page on the Web was nothing but a collection of static images, text, and links to other pages.
To leverage the simplicity of the Web paradigm, while enabling it to become a platform for the interactive applications that our users would need to use to access our knowledgebase, the VEP team created a new kind of web browser that could embed interactive applications directly into web pages, in a safe and secure way, thereby enabling it to become a universal interface to the entire VEP information system.
Still, there was the problem of the sheer size of the VEP datasets, and the computational complexity required to allow users to interactively explore the data. It was immediately clear that the PCs of the early ‘90s, thousands of times less powerful than those of today, would have no hope of being able to handle the enormous embryo datasets that our site would provide.
To solve this problem, we created a new kind of web system, using a widely distributed architecture, so that a small piece of a large distributed application would run within the web page, and that would tap into vast computational server capabilities transparently located remotely across the web. Today, of course, this kind of architecture is called “the cloud.” The VEP team demonstrated the first web-cloud application platform, with a 3-dimensional volume reconstruction of a 7-week-old human embryo embedded within a webpage, with the real-time dynamic visualizations being generated by a remote “cloud” of distributed computational engines and streamed to the browser, onstage to a stunned audience of early web pioneers at Xerox PARC in November of 1993. The cloud was an essential resource to enable the birth of spatial biology in the 1990s, and it continues to be so today. No matter how much compute power you can put on your desk, you will always be able to accomplish more, do higher-resolution reconstructions, do more intricate analyses, and answer bigger questions, by tapping into the vast computational and knowledge resources that the cloud allows.
The Advent of Single-Cell Spatial Genomics
NSTG: What do you think of the advent of single-cell spatial genomics which allows you to profile gene and protein expression in single cells and subcellularly in a tissue section?
Mike Doyle: I think it’s extremely exciting to see the emergence of systems like NanoString’s CosMx™ Spatial Molecular Imager (SMI) that break through the cell-resolution barrier to enable spatial imaging of subcellular phenomena. Being able to localize genomic activity at the organelle level will catalyze an explosion of new research projects looking to answer even more detailed questions about the dynamics of biological function.
NSTG: What do you think will be the challenges moving forward for the field of spatial genomics?
Mike Doyle: One of the challenges will be to extend the dimensionality of analyses into the third and fourth dimensions. While current projects are focused on two-dimensional sections, I believe it is inevitable that investigators will begin looking further into the third spatial dimension, and to dynamic changes in the temporal dimension, to create even more complete pictures of multicellular and intracellular structure and function.
The Future of Spatial Genomics
NSTG: What do you think lies ahead for the field of spatial genomics?
Mike Doyle: I believe that progress in the field of spatial genomics will only accelerate, and that it will enable important new discoveries across an increasingly wide range of areas of biology. I’m particularly intrigued by the parallel development of the field of spatial analysis, and I think there will be new breakthroughs in mathematics, statistics, and computational approaches for the characterization, analysis, and modeling of spatial phenomena not only in biology, but also in other fields rich with spatial data, such as geology, hydrology, and astrophysics, creating a new discipline that will move all these fields forward.
If you would like to learn more about how NanoString is helping to advance the field of spatial genomics, check out our website for more information on the GeoMx DSP, the CosMx SMI, and the AtoMx™ Spatial Informatics Platform, which provides scalable computing and storage space in the cloud for analysis and collaboration of spatial biology data from both GeoMx and CosMx.
For Research Use Only. Not for use in diagnostic procedures.