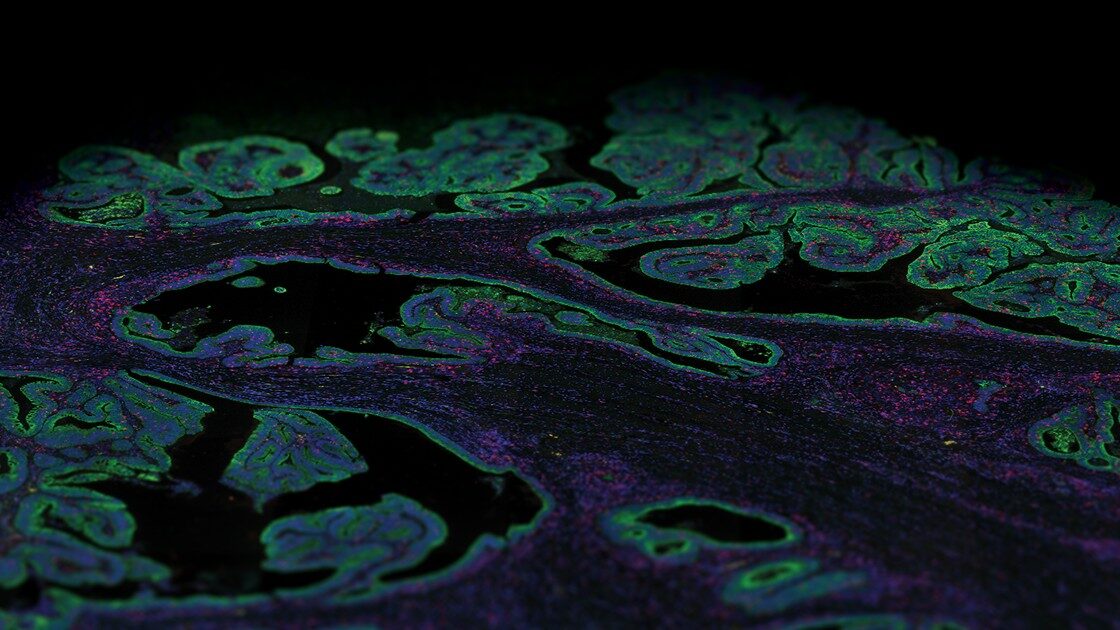
What does nTPM mean in the protein atlas?
The goal of the Human Protein Atlas (HPA) project is to map all human proteins in cells, tissues, and organs.
Further, it aims to annotate the function and spatial distribution of all the genes and proteins. To build a multi-dimensional spatiotemporal map of the human body, the Human Protein Atlas project uses an integrated omics approach combining multiple technologies for mRNA and protein detection including antibody-based imaging, transcriptomics methods such as scRNA-seq, and mass spectrometry. The HPA was initiated in 2003 and launched the first version of the public database in 2005. Since then, the human protein atlas gets updated regularly with more data and new website functionalities, and one of recent milestones consists of the addition of transcriptomics data based on high-throughput mRNA sequencing.
Single-cell RNA sequencing (scRNA-seq) and nTPM
Over the past decade much discovery and innovation in transcriptomics, research has been fueled by RNA sequencing (RNA-seq) approach that can detect and quantify mRNA transcripts from tissue samples. Further innovation to RNA-seq technology has been single-cell RNA-seq (scRNA-seq) which permits the comparison of the transcriptomes of individual cells.
Average TPM is equal to 106 (1 million) divided by the number of annotated transcripts in each annotation.
A major use of scRNA-seq approach has been to assess transcriptional differences within a population of cells or identify rare cell populations within a pool of cells. While quantifying the levels of expression for a given RNA sample, the nTPM (transcripts per million) value represents the number of transcripts detected for a given gene. The average TPM is equal to 106 (1 million) divided by the number of annotated transcripts in each annotation and thus is a constant.
Single-cell resolution of transcriptomics data
To increase the resolution at a single-cell level, three types of gene expression data have been integrated into the human protein atlas namely- single-cell RNA-seq, immunohistochemistry, and deconvolution analysis. The recent version 22 uses transcriptomics data to classify genes into clusters. Raw expression data of scRNA-seq from each tissue sample are grouped into clusters based on their expression across cells, tissues, and organs. The main cell type of each cluster is identified through manual annotation based on deconvolution methods and immunohistochemistry expression data. In bulk RNA seq data, the deconvolution method estimates the fraction of a cell type from samples composed of multiple cell types.
This method takes advantage of the fact that there are genes that are particularly expressed by a single cell type in a regular manner (reference genes) and thus reflecting the quantity of that cell type in a tissue sample. Hence, the expression levels of the reference genes are compared to the expression levels of all other genes across all tissue samples to determine the degree of correlation (0–1), creating a surrogate measurement of the correlation between specific cell types and all protein-coding genes. For genes with low expression levels, expression data within cell type clusters are pooled to generate normalized transcript per million (nTPM) for each gene and cell type.
Future directions
The Human Protein Atlas gets updated regularly based on careful curation of the data. Curation involves, the removal of data/antibodies/images which do not meet the database’s quality criteria. Subsequently, new improved versions of the data/antibody/images are replaced when possible.
The last few years have seen the emergence of several spatial technologies that can quantify and detect protein and mRNA expression levels to understand localized transcriptional changes while maintaining information on tissue architecture. The multiomics high-plex spatial technologies by NanoString, such as the GeoMx® Digital Spatial Profiler and the CosMx™ Spatial Molecular Imager, which are compatible to work on archival FFPE preserved tissue (a valuable resource for RNA) and proteins, are providing unprecedented insights into the spatial organization of tissues and how fundamental cellular processes are orchestrated in multicellular organisms.
The CosMx™ SMI and decoder probes are not offered and/or delivered to the Federal Republic of Germany for use in the Federal Republic of Germany for the detection of cellular RNA, messenger RNA, microRNA, ribosomal RNA and any combinations thereof in a method used in fluorescence in situ hybridization for detecting a plurality of analytes in a sample without the consent of the President and Fellows of Harvard College (Harvard Corporation) as owner of the German part of EP 2 794 928 B1. The use for the detection of cellular RNA, messenger RNA, microRNA, ribosomal RNA and any combinations thereof is prohibited without the consent of the President and Fellows of Harvard College (Harvard Corporation).
The GeoMx DSP excels at unbiased biomarker discovery through its whole transcriptome profiling capabilities.
The GeoMx DSP and CosMx SMI perform complementary functions. The GeoMx DSP can profile 18,000+ protein-coding genes while maintaining a wide dynamic range for the detection of low to high-expressing genes, plus over 150 proteins for a high-plex, spatial multiomic solution. Thus, the GeoMx DSP excels at unbiased biomarker discovery through its whole transcriptome profiling capabilities and has been instrumental in generating a collection of normal human tissues, the Spatial Organ Atlas, of six different human organs: kidney, brain, colon, liver, lymph node, and pancreas. The CosMx SMI, on the other hand, facilitates spatial biology at the single-cell and subcellular levels with the ability to detect more than 1,000 RNA and over 64 protein analytes. Therefore, CosMx SMI is perfect for creating a spatial cell atlas, mapping cell types, and examining cellular interactions.
As the human protein atlas continues to evolve, the application of these multiomics platforms will enable high-throughput profiling of genes including capture variance and heterogeneity in a large population, contributing to a more in depth understanding of biological mechanisms and disease.